In data-driven organizations, decision-makers, be it a marketing manager or even a C-level executive, expect numbers and solid proof that a suggested change will work. This is because changing a key page or app element that's working well can easily kill your company's sales and revenue.
That’s why A/B tests have become so popular and are performed routinely before some changes are made to the functioning and appearance of a landing page, website application, website design, or even email campaign.
When starting your journey with A/B tests, there is some learning curve you have to account for. Don’t expect to become an A/B testing guru after just a couple of tests!
On your way, you will probably make dozens of mistakes and your A/B tests will fail or bring wrong results. That's something you can’t avoid and, at the same time, this is a hard truth that decision-makers (and your boss) don’t want to hear!
Don’t worry as we got you covered with some most common mistakes in A/B testing in this article. We will also explain what you should do to prevent them if you are about to conduct your first A/B tests.
Neglecting statistical significance
Statistical significance shows the likelihood that the difference between your experiment’s control and test versions is caused by random chance or error.
If you don’t pay attention to statistical significance and rely on your gut feeling, not on calculations, you are likely to end your test too early, before it generates statistically significant results. This also means that you will get the wrong results.
Statistical significance is subject to a simple calculation – you don’t have to hold a degree in economics or science to perform it. On the Internet, you have a wide choice of free statistical significance calculators that will help you estimate what is needed to achieve it.
Here are some of the variables that such calculators take into account:
- Number of visitors in a control version
- Number of visitors in a test version
- Number of conversions in a control version
- Number of conversions in a test version
You just pull this data in and get an answer if your results will be reliable with your current traffic and conversion volume.

You can also review a chart and analyze calculations in more detail as most calculators offer some sort of results visualization.
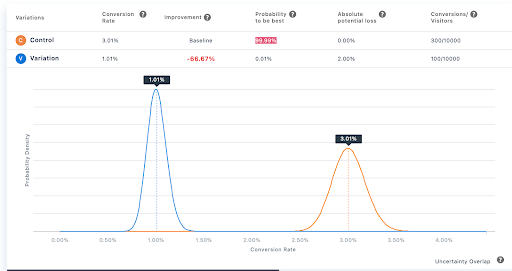
Key takeaway – Don’t make an assumption stating that a typical experiment should last around two weeks. Instead, calculate how much traffic and conversions you need (both experiment and control versions) to make sure your test has statistical significance. Use online calculators or other collaboration tools to evaluate, plan, launch, and assess A/B tests together with your team.
Testing too many things at once
It’s tempting to change many page elements at once – for example, using different email personalization, adding emojis, company names in email subject content, and more all in the same test.
Saving time on lengthy tests is one of the reasons why people choose to test many different things at once. However, if you go with this strategy, you might not understand what worked in your experiment and what didn’t. With the example mentioned above, you can improve your email deliverability, open rate, and conversions without knowing what change has made a difference on those metrics.
If you need to test more than one element on the page, consider using multivariate testing and only with the websites that have decent traffic to test various test versions at once. By using this technique, you can test your new images, check the performance of different title tags, and sign-up form length – all under separate test versions.
Multivariate testing is a technique that helps test a hypothesis in which various variables are changed.
Key takeaway – Don’t set up multiple split tests running at the same time. Instead of split tests, consider using multivariate testing. Testing a few things at once will only work with high-traffic websites.
Choosing a wrong hypothesis
When launching A/B tests, you should start with formulating a hypothesis that states a problem and a solution to it, as well as explaining key success metrics to measure results. Your hypothesis can’t be based on random things – otherwise, running an A/B test won’t take you anywhere.
A hypothesis is an explanation or solution to a problem. For example, you could discover that your users don’t trust your website because it doesn’t look like a trustworthy business to them and that’s why they decide not to buy. You consider it as a problem to solve. By adding some trust badges on your website, you aim at boosting your visitors' trust which should result in a higher conversion rate.
Base your hypothesis on the evidence you collect from users. Your test’s goal should be resolving a specific problem that users face. You usually discover problems by observing user behavior or talking to them.
Remember that measuring results is also a critical element of a hypothesis. That’s why choosing the right success metrics to measure before and after the test is key to your success.
Key takeaway – Base your hypothesis on a real problem users face and identify a solution that can eliminate this problem. The right hypothesis is based on evidence, not assumptions about it.
Testing meaningless things
There are obvious things and well-known facts. Do they have to be tested a hundred times to prove they work? They definitely should not, because it’s a waste of time.
Take an example of signing up your SaaS users for a trial version of a paid account instead of a freemium version. If you change a button URL to sign up users for the paid version and hide a mention about a forever free account (on which users can stay without paying after their trial ends), there is nothing to test here. Users don’t have a choice – they will all be automatically signed to a paid version.
This seems pretty obvious, right? There is no way users would dig through every single page of your website looking for a forever-free plan if you don’t communicate it on your pricing page.
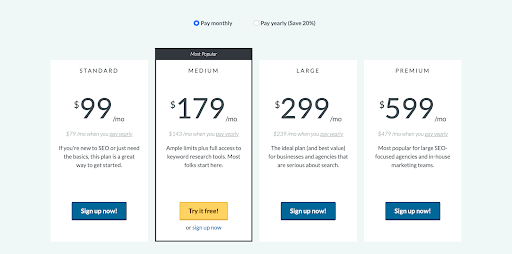
Key takeaway – Don’t waste your time on testing obvious things including ad creatives that hardly differ from each other. Focus on testing page elements that can have a tremendous impact on your conversion rate. It all goes down to better prioritization.
Neglecting traffic
A/B tests are not the right tool to use for small websites with very little traffic. By setting up experiments on a website attracting 200 visitors every month and only a handful of conversions, you would need months if not years to achieve statistical significance and become certain that the test results are true and are not due to a chance or mistake.
You should evaluate the traffic needed to run a standard test before you launch it. Otherwise, running A/B tests won’t take you anywhere – you will only waste time and money on set-up and observation. In this situation, you might want to focus on driving more traffic to a website using Google Ads and other relevant channels, so your tests can achieve statistical significance faster.
Key takeaway – Calculate how much traffic you will need to run a test achieving statistical significance before you launch it. Don’t use A/B testing if it takes months or years to achieve statistical significance.
Giving up on tests
If you have failed your test, you shouldn’t stop with your A/B testing routine. Even though it’s easy to give up if you don’t see instant results that impress everyone, it shouldn’t be a reason for you to stop testing. However, you should create a report after every A/B test that has failed.
Take some time to think and look for reasons. For example, your new landing page test could have failed, because you could do many things wrong:
- Using wrong images that don’t resonate with your audience (e.g. using images of students for an audience where the average age is 35+, using too many unnatural stock images).
- Forgetting about page responsiveness (you have a big chunk of mobile traffic that lands on a website that is poorly optimized for mobile devices).
- Using data collection forms with too many fields.
- Not communicating your unique selling point and benefits of using a product in your landing page copy.
There are many other things that you can do wrong. To identify them after your test has failed, you need to do proper brainstorming and consider all possible reasons for failure.
Next step would be coming up with a new hypothesis for each test and starting again. For example, you might decide to work on improving page speed for users accessing websites from mobile devices or, if your company is large enough, even hiring a site reliability engineer for this and other tech tasks.
Key takeaway – don’t give up on A/B testing too early. Always summarize your test results and think of what could be a reason for failure. Come up with a plan for your next A/B test to fix it.
Wrapping up
There are many reasons for A/B tests to fail. If you have just started your advantage with this testing method, there are many areas where you can make mistakes. Making mistakes in the beginning can be quite disappointing and even get you to quit A/B testing before you even see the first results.
You shouldn’t give up too early. In this article, we have mentioned the most common mistakes beginners make when starting with A/B testing and explained the ways to prevent those mistakes from happening.
Let’s summarize it!
- Keep in mind statistical significance and use online calculators to understand how long you should run your tests to be sure they are not due to error or chance.
- Use split testing to test only one-page element change. It’s always better to test one thing at a time. However, if your website attracts a lot of traffic every month, you can also experiment with multivariate testing.
- Base your hypothesis on evidence and some problem your users face. Aim to resolve it with an action followed in an A/B test. Don’t waste time on testing random things.
- Test things that matter the most and can have a huge impact on your business bottom line. Don’t waste time on testing facts or obvious things.
- Calculate how much traffic you need to achieve statistical significance before you kick off your test. A/B testing might not be the right tool for websites with low conversion volume and traffic.
- Don’t give up too early. Come up with a summary of what could cause failure and make a plan to change it.